Introducing Tiny-LLM: A Comprehensive Guide to LLM Serving
In the rapidly evolving landscape of machine learning, a new project called Tiny-LLM has emerged, aimed at providing a practical tutorial for system engineers on how to efficiently serve large language models (LLMs). Currently in its early stages of development, Tiny-LLM is an ambitious effort that utilizes the MLX framework to facilitate deep learning processes without relying on high-level neural network APIs.
The primary objective of Tiny-LLM is to equip users with essential techniques and practical knowledge necessary for serving a large language model, particularly focusing on the Qwen2 models. These models are significant in the field of AI because they demonstrate the capabilities of LLMs in understanding and generating human-like text.
One of the reasons MLX was chosen for this project is its accessibility. In todays tech environment, establishing a local development environment on macOS has become more straightforward compared to the complexities associated with setting up an NVIDIA GPU. This democratization of access allows more individuals to experiment with and learn about machine learning without requiring expensive hardware.
Why Qwen2, in particular? For many, including the creator of Tiny-LLM, Qwen2 was their first interaction with large language models. It has become the standard example referenced in documentation for vllm, a framework that aids in the efficient training and serving of large language models. The creator has invested significant time analyzing the vllm source code, gaining insights that have informed the development of this tutorial.
For those who are interested in diving deeper into the subject, a comprehensive guide titled
Hans Schneider
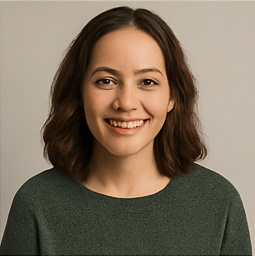
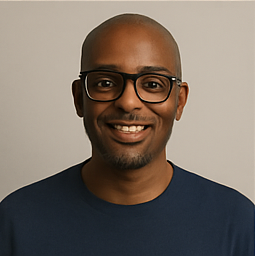
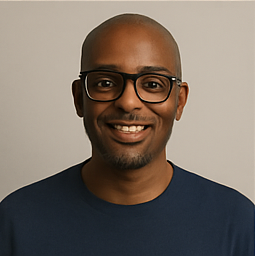
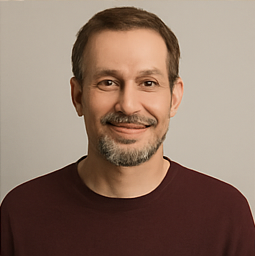
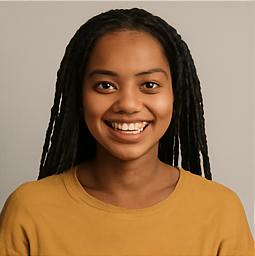
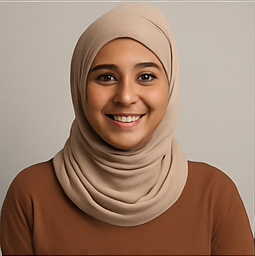
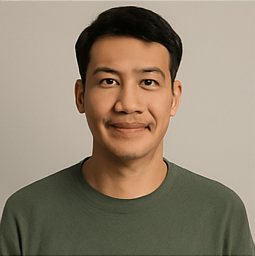
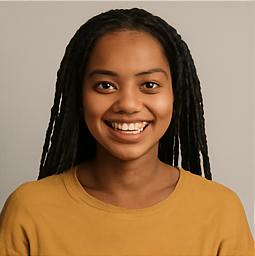
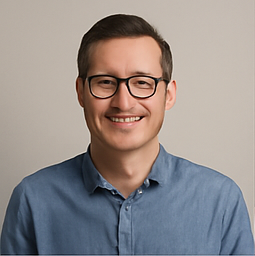
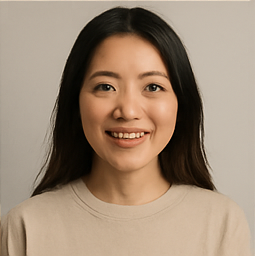
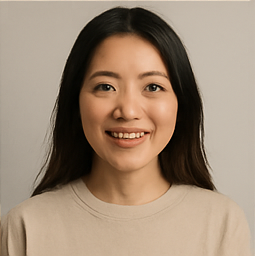