Exploring Communication Models in Brain Networks: New Insights into Optimal Influence
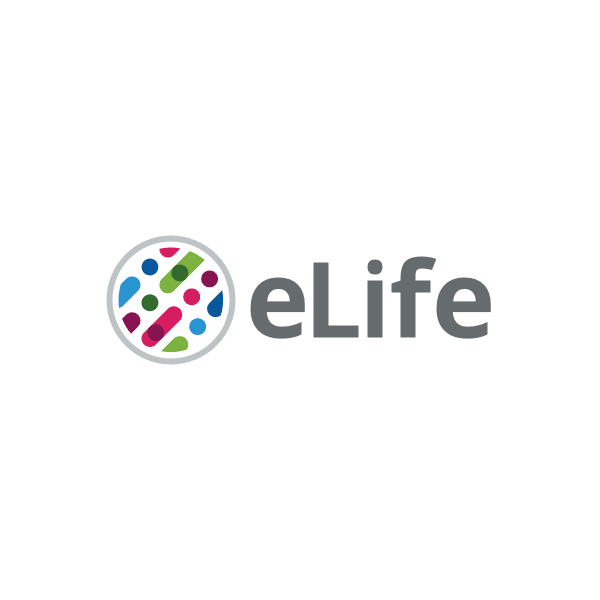
In a recent study, researchers sought to elucidate the most accurate models of signal propagation that represent the complex indirect influences between various regions of the brain. The study, led by Seguin et al. (2023, 2020), examined a range of communication models, from routing strategies to diffusion processes, to better understand how information travels within neural networks.
Routing strategies suggest that signals predominantly travel along the shortest possible paths. One of the simplest forms of this concept is embodied by the Shortest Path Efficiency (SPE), which focuses solely on the most direct routes. In contrast, Navigation Efficiency (NE) is a more sophisticated model that minimizes physical distance to a target node at each step of the journey. Another measure, called Search Information (SI), quantifies the amount of information necessary for a random walker to successfully navigate the shortest path between two points. A higher information requirement implies more significant communication challenges between nodes.
On the opposite end of the communication spectrum are diffusion processes, which envision a cascading flow of information throughout the network. This approach allows for longer, sometimes recurrent routes to reach the same nodes. The efficiency of signaling within these processes is evaluated using two primary metrics: Communicability (CO) and Diffusion Efficiency (DE). CO operates through a broadcasting strategy, where information spreads from the source and reaches the target through several alternative pathways, albeit with an exponential decay at each step. This decay reduces the influence of longer pathways. DE, however, does not account for such attenuation; it simply counts the steps taken by a random walker from the source to the target.
To quantify the relationships between these models and optimal influence (OI), a pairwise Pearsons correlation was conducted. The findings revealed a substantial positive correlation between OI and CO (R=0.88), while a significant negative relationship was identified with SI (R=0.68). Other metrics like SPE, NE, and DE exhibited more complex, nonlinear relationships with OI. This suggests that while all communication models possess some capacity for capturing OI, the model that embraces an attenuated diffusion dynamic is particularly effective. This aligns with previous findings that indicate COs capability in representing the spread of electrical stimulation to previously unconnected nodes (Seguin et al., 2022a) and in accurately predicting large-scale functional modules (Seguin et al., 2022b).
However, researchers also observed that CO tends to slightly underestimate the influence of longer pathways. To address this limitation, they explored three alternative approaches: scaling communicability with a factor (scaled communicability), utilizing a linear rather than exponential attenuation (Linear Attenuation Model; LAM), and employing a covariance structure from a spatial autoregressive model (SAR). Each of these models incorporates a single adjustable parameter: a scaling factor for scaled CO, an attenuation factor for LAM, and a degree of spatial influence for SAR. After calibrating these parameters to replicate OI, it was found that only the SAR model significantly improved performance (R=0.997), while scaled CO and LAM provided minor enhancements (R=0.89 and 0.90 respectively).
The SAR model outperformed CO by revealing that CO is overly rigid in discounting longer pathways. This discrepancy highlights that CO assumes signals fade drastically faster than they actually do, indicating a skewed positioning on the spectrum of signal propagation dynamics.
Further analysis investigated the influence depth, characterized by the number of hops a signal can make before its impact is significantly diminished. Utilizing a linear regression model, researchers predicted OI from progressively diffused signals. Results indicated that the optimal predictive performance occurred at the sixth hop, achieving an R of 0.75, meaning the first six steps accounted for 75% of the variance in OI. A multivariate, regularized Lasso regression model identified that walks of lengths five, six, and eight contributed most significantly to predicting OI with high accuracy (R=0.96).
Despite the network diameter being around ten steps, the findings suggested that information flow between the most distant nodes is considerably degraded after approximately eight steps. The superior predictive capabilities of the SAR, LAM, and CO models further emphasized the complexity of communication dynamics that extend beyond the initial processing pathways.
Ultimately, the study revealed that while SAR and other communication models have their limitations in accurately characterizing optimal signal propagation in larger, directed networks, the influence of brain regions often extends further than traditional models suggest. The enhanced performance observed from mouse to macaque connectomes indicates the role of network size and complexity in shaping these dynamics.
In conclusion, these findings underscore the necessity of employing more nuanced communication models that embrace the complexities of brain network dynamics, especially as linear models may overlook the rich, nonlinear operations and oscillatory behaviors inherent to cognitive processes. Future research should aim to validate these insights against realistic dynamics that reflect the multifaceted nature of brain activity.