Scholars Gather at Hoover Institution to Address AI's Political Bias and Trust Issues
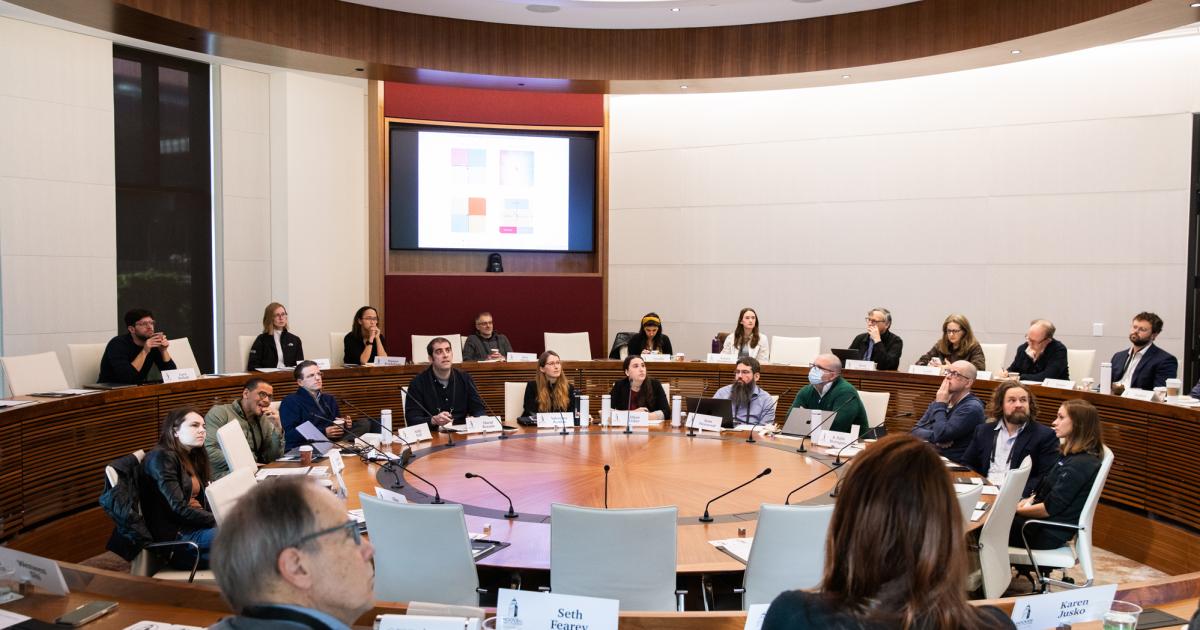
The Hoover Institution, located at Stanford University in California, recently hosted a pivotal gathering to address the growing influence of artificial intelligence (AI) on public opinion and the dissemination of information. As AI continues to play a significant role in shaping societal narratives, scholars convened on March 21, 2025, to explore how generative AI models can be developed to earn the trust of all Americans amidst an increasingly polarized political landscape.
Andrew B. Hall, a Senior Fellow at the Hoover Institution, organized the conference with the goal of discussing how future AI models and agents can embody values that resonate with the public. Hall emphasized the pressing need for these technologies to reflect collective societal values while highlighting the potential for companies and governments to unintentionally or deliberately instill specific ideological biases in these models.
This conference was sponsored by Hoovers Center for Revitalizing American Institutions (RAI) and is part of a broader initiative to counteract the declining public trust in institutions and bridge the widening divide between the extremes of Americas political spectrum.
To frame the challenges posed by AIs political biases, Hall encouraged participants to assess the political leanings of leading AI models. David Rozado, an associate professor from Otago Polytechnic in New Zealand, presented notable findings from his research indicating that many prominent large language models (LLMs) tend to lean left politically. Rozado pointed out that these AI systems frequently reference left-wing figures more often than their right-wing counterparts, raising concerns about potential bias in AI-generated content.
In an attempt to address this issue, Rozado introduced three new LLMs: one that leans right, another that leans left, and a third model dubbed depolarizing GPT, which is engineered to provide centrist responses to political inquiries. This innovative approach seeks to create a more balanced dialogue in AI interactions.
The implications of politically slanted AI tools are becoming increasingly evident. Jillian Fisher from the University of Washington shared research findings from a survey involving 300 participants who engaged with the three different LLMs without prior knowledge of their political biases. The results revealed that the model with a clear political slant effectively swayed the opinions of participants affiliated with either the Democratic or Republican parties on certain issues.
Conversely, those who demonstrated a higher-than-average interest in or understanding of large language models were less susceptible to opinion changes after interacting with the AI. This suggests that awareness of AI functionalities may act as a buffer against ideological shifts.
During the conference, industry representatives discussed the strategies they employ to create LLMs that minimize the risk of ideological bias. However, many highlighted the considerable challenges inherent in achieving such neutrality. There was a consensus among participants that a government-mandated approach to enforce political neutrality in AI models would not be feasible, given the multitude of complexities involved.
The subjective nature of defining political neutrality was emphasized by several academics throughout the day. Questions arose about who would be responsible for overseeing the content generated by AI models and determining what constitutes a neutral stance. As the discussion evolved, a vision emerged of a marketplace featuring diverse LLMs, each reflecting different political perspectives. Employing research-based techniques to measure the political slant of these models could enable companies to present users with a selection of AI systems tailored to their values, although this approach risks creating echo chambers.
Additionally, the potential for restrictions on certain AI models based on their country of origin was raised. The DeepSeek R1 model, developed in China without utilizing advanced general processing units (GPUs) and produced at a significantly lower cost than comparable U.S. models, is expected to face bans or stringent restrictions within the United States and allied nations in the foreseeable future. Some participants noted that this could be a positive outcome, given that DeepSeek models have shown tendencies to avoid discussing sensitive topics, such as the Tiananmen Square massacre and the mass internment of Uighurs in China.
The challenge of achieving complete political neutrality in AI models is further complicated by the inherent subjectivity in various developmental aspects. Sean Westwood, a visiting fellow at Hoover, described this concept as a moving target, showing that users perceptions of LLMs political slants often vary based on their individual ideologies and the subjects under discussion.
Valentina Pyatkin from Seattles Allen Institute for AI demonstrated that many leading models tend to provide ambiguous responses or refuse to answer political questions altogether unless explicitly directed to do so.
Legal perspectives were also examined, with Senior Fellow Eugene Volokh highlighting the difficulties of using law to enforce neutrality among AI models. He argued that any legislative efforts aiming to impose neutrality could potentially violate First Amendment rights. Furthermore, he cited ongoing legal cases in the U.S. where LLMs were involved in libelous claims, such as an accusation against a Georgia gun rights activist of embezzlement, which was a complete fabrication.
Interestingly, public sentiment appears to diverge when it comes to supporting legal protections for AI-generated content compared to human speech. Jacob Mchangama from the Future of Free Speech Project at Vanderbilt University reported that surveys indicate a lower tolerance for controversial content produced by AI than by humans. This shift in attitudes towards free speech in the U.S. has been highlighted by a recent survey, revealing declining support for free speech over the past four years, which may influence how regulations surrounding free speech and AI content evolve.
The conclusion of the conference featured a spirited discussion regarding the need for a balanced approach to prevent a future dominated by a few AI models that dictate socially acceptable ideological views. Alice Siu from Stanfords Deliberative Democracy Lab and Bailey Flanigan from MIT emphasized the importance of involving users in decision-making processes concerning the tech platforms they use, advocating for a user-centric approach to navigate controversial and value-laden issues.
As the future of AI remains uncertain, the implications of ideologically misaligned LLMs for users and society at large are profound. Nevertheless, this conference served as a crucial forum for fostering evidence-based policies aimed at promoting ideological diversity among AI models without infringing upon legal principles of free speech or privileging certain viewpoints over others.