Groundbreaking RNA Sequencing Analysis of Staphylococcus aureus Unveiled
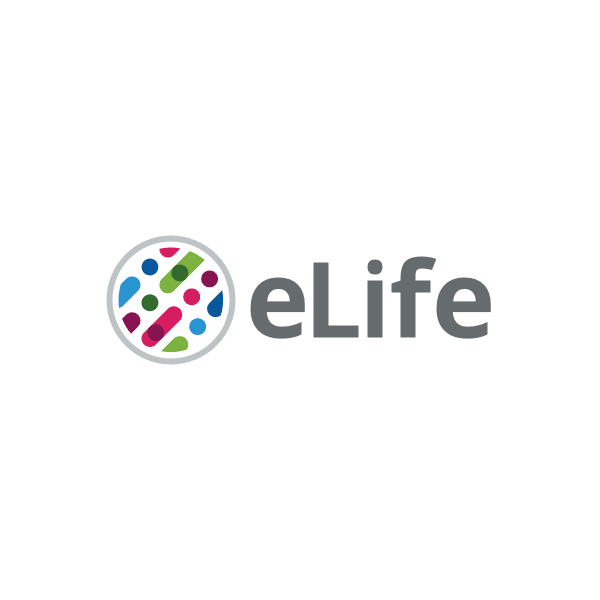
In a remarkable leap forward in the field of microbiology, researchers have harnessed the power of the pymodulon package (Sastry et al., 2021) to perform an Independent Component Analysis (ICA) on diverse strains of Staphylococcus aureus. This thorough investigation involved the systematic acquisition of all accessible RNA-sequencing data for both the non-USA300 and USA300 strains, which were subsequently processed through a stringent Quality Control/Quality Assurance (QC/QA) pipeline. The research team meticulously curated the data to enhance metadata accuracy and ensured alignment with the TCH1516 genome, corresponding to the accession numbers NC_010079, NC_012417, and NC_010063.
A crucial element of this research was the transformation of the aggregated RNA sequencing data into log-TPM (transcripts per million) format, normalizing it to a single reference condition. The selected reference conditions included SRX3760886 and SRX3760891, standing apart from other ICA models that typically rely on project-specific reference conditions. This alternative normalization method can inadvertently obscure vital strain-specific information, particularly since many BioProjects feature data derived from only one isolate, such as NCTC8325, TCH1516, or LAC.
Following the established methodological pipeline, the ICA was executed to generate iModulons specifically for the CC8 clade of S. aureus (Sastry et al., 2019). This process commenced with the gathering of all relevant RNA-sequencing data and associated metadata for CC8 strains, predominantly spotlighting well-known strains like TCH1516, FPR3757, LAC, Newman, and NCTC8325. While some samples were less precisely categorized, identified simply as USA300, they nonetheless belonged to the CC8 clade.
The fastq files extracted from these samples underwent a trimming process utilizing TrimGalore (v0.6.5) before being aligned to the reference genome of TCH1516 via bowtie2 (v1.2.3) (Krueger, 2015; Langmead and Salzberg, 2012). The gene-specific read counts were subsequently computed using HTSeqCount (v2.0.1), applying strict intersection criteria to ensure high accuracy. Following this, the number of mapped reads was normalized to TPM and log-transformed into log-TPM.
Before the data could be utilized for further analysis, the quality of reads and alignment was rigorously examined using FastQC and MultiQC (v 1.11) (Andrews, 2010; Ewels et al., 2016). Samples that failed to meet the stringent criteria for per base sequence quality, per sequence quality score, per base n content, or adapter content were excluded from the analysis. Additionally, any samples with fewer than 500,000 reads aligned to the reference genome were omitted. The final selection process was meticulous, as samples lacking replicates, or those with replicates displaying Pearson correlation coefficients lower than 0.9, were also excluded. Ultimately, additional metadata for the remaining 670 RNA-sequencing samples was compiled, encompassing crucial aspects such as growth conditions and genetic alterations.
To facilitate comprehensive analysis, the log-TPM data were centered around the reference condition of S. aureus TCH1516 cultured in RPMI+10% LB. This centering process enabled ICA to effectively capture strain-specific regulatory changes. For instance, it underscored the activity of the Fur transcription factor, depicted as a linear combination of the Fur iModulon, which comprises genes regulated by Fur, alongside a secondary strain-specific iModulon that delineates the differences between USA300 and non-USA300 strains.
Subsequently, FastICA was applied to the centered log-TPM data, which resulted in the computation of the M and A matrices, essential for defining the structures and respective activities of the iModulons (Pedregosa, 2011; Koldovsk et al., 2006). Determining the optimal number of stable components was a critical aspect, as FastICA is a non-deterministic method; thus, multiple iterations could yield varied component weightings and activity levels. Moreover, spurious components could emerge, which only appeared inconsistently across a subset of runs.
In order to ensure stability, ICA was executed a total of 100 times with a random seed. Similar components, such as those encompassing Fur-associated iModulons, were clustered using the DBSCAN algorithm to identify consistent patterns over various iterations. Only components that were present in every run were accepted for the final analysis. During the ICA process, researchers had to specify the number of components into which the data would be decomposed. Selecting too few components could result in signals from multiple transcription factors merging into a single component, whereas excessive decomposition might lead to numerous unstable single-gene iModulons that merely reflect noise within the dataset.
To pinpoint the optimal number of components, the heuristic method OptICA was employed. This innovative method runs ICA with varying numbers of input components, ranging from 10 to 340, ultimately recommending an optimal number that minimizes single-gene iModulons while maximizing robust components (McConn et al., 2021). The final model was constructed with 270 input components, of which 148 were classified as robust.
The analysis of each component was thorough, and a gene was classified as part of an iModulon if its weighting did not conform to a Gaussian distribution, as determined by DAgostinos test. The genes associated with each iModulon were subsequently cross-referenced with genomic features such as regulons, phages, and mobile cassettes to identify significant overlaps (hypergeometric test; adjusted p-value <0.05, precision 0.5, and coverage 0.2). Furthermore, other iModulons linked to specific biological features, such as those involved in translation, were meticulously curated and labeled to reflect their properties accurately. The activities of the resulting iModulons were individually assessed to highlight those exhibiting the most pronounced strain-specific differences.