Advanced Classification Techniques in Nanopore Sequencing for Plasmid Analysis
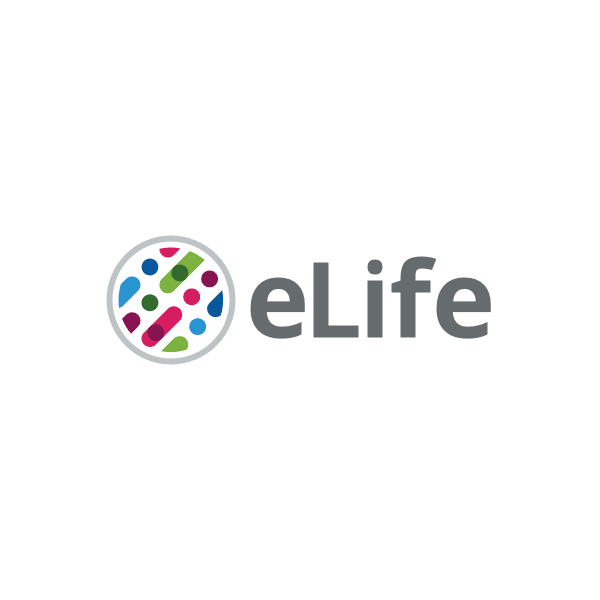
In a groundbreaking study on nanopore sequencing, researchers have developed advanced methods to classify reads from various plasmids using highly sophisticated algorithms. The initial step in this classification process involves aligning each read from the sequencing output against a reference plasmid, denoted as pi, and the corresponding query sequence, qk. This alignment utilizes parameters that have been previously established in the pre-survey algorithm, ensuring consistency and reliability in the results.
To systematically evaluate the alignment, researchers calculate a normalized alignment score denoted as api, qk, achieved by dividing the alignment score by the length of the reference plasmid, pi. Subsequently, the plasmid assignment for each query is determined as follows:
pqk = argminpi P api, qk,
where P represents the collection of reference plasmids involved in the analysis. This rigorous process defines the assigned plasmid, pqk, based on its alignment with the query qk. Notably, if the normalized alignment score is below a pre-defined cutoff, referred to as score_threshold, the read is excluded from further analysis. This threshold is crucial for filtering out short reads that may compromise the quality of subsequent data interpretation.
Moreover, any read qk that demonstrates the same normalized alignment score across multiple plasmids is omitted. Such instances indicate that the read lacks sufficient distinctive information to accurately assign it to a specific plasmid. Additionally, any read that is over twice the length of the assigned reference plasmid, and yields a normalized alignment score greater than 1, is also excluded to eliminate potential ambiguities stemming from plasmid multimers.
The study presents findings from multiplex sequencing involving six distinct plasmids characterized by moderate similarities. Figures 3 and 4 illustrate the results from these experiments. Group I showcases multiplex sequencing of six plasmids that exhibit only modest similarities, whereas Groups II through IV focus on pairs of plasmids that are closely related.
Figure 3a displays the pre-survey outcomes for Group I, revealing the emergence of two primary clusters: one consisting of four similar plasmids (P1P4) and another comprising two (P5P6). These clusters exemplify plasmids that, while sharing a common vector backbone, possess different inserts. This moderate similarity makes them amenable for multiplex sequencing, supported by the distance metrics indicating that the clustering distances are notably greater than 20, a benchmark deemed safe for quality analysis.
The nanopore sequencing method employed here allows for an efficient analysis of six plasmids mixed and assessed as a single sample. Figure 3b details the general quality metrics of the sequenced reads, illustrating distributions of read lengths and quality scores, which are essential for evaluating the sequencing performance.
Furthermore, detailed scatter plots of the normalized alignment scores are depicted in Figure 3c. These plots are instrumental in determining the score_threshold value, as they provide insights into how adjustments can improve classification accuracy. The data indicates that while a higher threshold enhances accuracy, it simultaneously reduces the number of reads assigned to each plasmid. Generally, the threshold of 0.5 has proven to be effective, but the researchers emphasize the importance of user customization based on specific experimental conditions.
In experiments involving closely related plasmids, a significant concern arises regarding the accurate classification of reads. Theoretically, the classification of reads depends heavily on the unique sequence differences among the plasmids. The results depict that even small variations between plasmids can lead to reliable classifications due to the high accuracy levels achieved with recent nanopore sequencing technologies.
The team conducted a quantitative analysis on three sets of closely related plasmids, where they utilized Levenshtein distances of 1, 2, and 3 to assess classification accuracy. Results indicated that despite the close similarities, most reads could be accurately classified, as shown in Figures 4df. Although the data points largely overlapped the line where y=x, a closer examination in the magnified views revealed clear deviations indicating successful differentiation between the closely related plasmids.
Moreover, the study employed a simple fitting method to evaluate the rates of incorrect classifications in the dataset. By extracting reads that align with sequence-differing regions, the researchers exhibited that the classification accuracy remains impressive even at low error rates, which were further reduced with higher Levenshtein distances.
Following the classification phase, each read undergoes alignment against its corresponding reference sequence, culminating in a comprehensive post-analysis that aims to generate a consensus sequence. This process employs Bayesian analysis techniques, integrating the quality scores of the reads and prior information on possible error rates during plasmid construction.
The consensus base call is derived by calculating probabilities for potential true bases, ultimately selecting the base call that demonstrates the highest likelihood based on the accumulated evidence. This method has been refined to accommodate the evolving accuracy of the quality scores associated with Oxford Nanopore Technologies' sequencing outputs.
Overall, this study showcases the effective integration of advanced computational techniques with nanopore sequencing, paving the way for enhanced plasmid analysis methodologies in genomic research.