Exploring the Intersection of Artificial Intelligence and Theoretical Physics
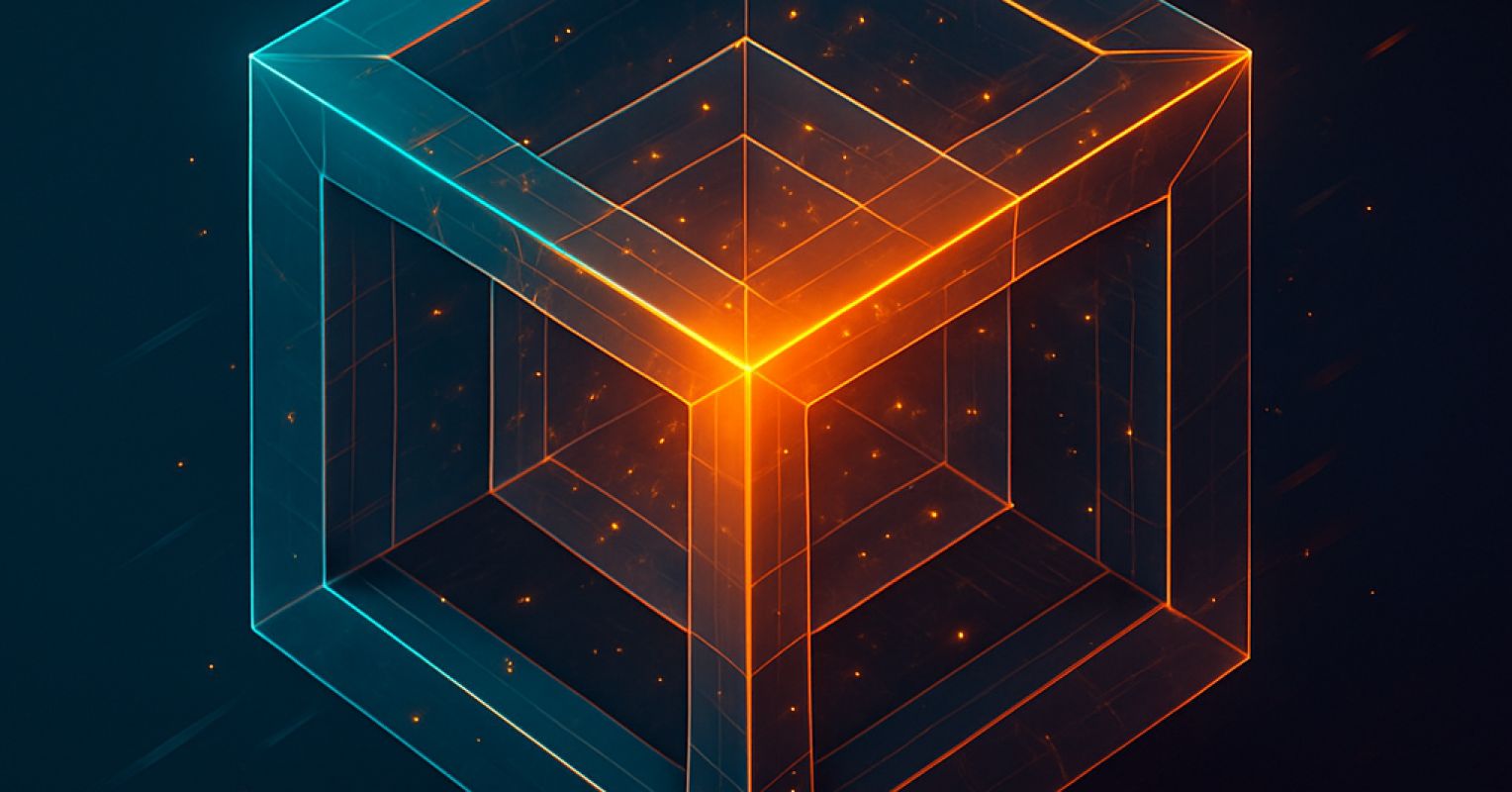
In my previous article titled Artificial Intelligence and the Shape of Reality, I ventured into a thought-provoking exploration that unexpectedly linked large language models (LLMs) with the enigmatic dimensions of string theory. This connection between two seemingly disparate fieldsmachine learning and theoretical physicsilluminated concepts that merit further investigation, revealing a profound intersection of ideas.
This article serves as a continuation of that inquirya Chapter 2 in this intellectual journey. Here, I aim to delve deeper into the structure of these dimensions and the functions that arise from them. Specifically, I will examine what occurs when these expansive spaces collapse into actionable units, such as a single word or an elementary charge.
Lets begin our exploration with a seemingly simple concept: the dot product.
From High Dimensions to a Single Word
In the realm of large language models, every word is represented not merely as a symbol but as a point within a vast multidimensional space. This space can extend to thousands of dimensions12,288 in certain modelswhere each dimension encapsulates a distinct aspect of meaning, context, or syntactic relationship. Unlike conventional spatial dimensions such as height or depth, these are abstract axes that reflect semantic relevance. Envision meaning itself materializing as a complex geometric structure.
When an LLM predicts the next word in a sentence, it engages in an operation that is surprisingly elegant. The model first considers the current context, which is also a vector in this high-dimensional space. It then compares this context vector to the vectors representing each possible next word. How does it accomplish this? The answer lies in the dot producta mathematical operation that effectively measures the alignment of two vectors.
Its important to note that this comparison isnt restricted to just a select few likely words; rather, its made against every token in the models extensive vocabulary, often comprising 50,000 or more words. The LLM executes the dot product calculation for each of these word vectors simultaneously, utilizing matrix operations. This process is neither linear nor sequential; it is geometric and operates in parallel.
To clarify further, this isnt the vector addition we often visualizewhere arrows are added tip to tail. Instead, its a comparison. The dot product takes each corresponding dimension of the two vectorsone representing context and the other the candidate wordmultiplies them together, and then sums all those products. The result is a scalar valuea single number that conveys magnitude but lacks direction. This aspect is crucial, as it indicates not where to go next but rather how strongly a specific word fits within the context at that moment. It distills a vast and intricate geometry into a singular score of relevance.
This scalarthis seemingly mundane numberis the basis upon which the model decides what comes next. The remarkable fluency exhibited by LLMs is fundamentally built on billions of these scalar reductions. In a very tangible sense, language emerges through projection, and projection results in reduction.
Shifting Gears: Electrons and Charge
If this appears abstract, lets transition to a more tangible realm of physics and consider the electron. The charge of an electron is regarded as a fundamental natural constant, often simplified to -1. However, within the context of quantum field theoryand more dramatically, string theorycharge isnt merely assigned; it emerges as a property of the underlying structure.
In string theory, particles are interpreted as different vibrational modes of minuscule strings that exist within a universe potentially comprising up to 11 dimensions. Many of these dimensions, as I noted previously, are compactifiedcurled into intricate shapes known as Calabi-Yau manifolds. The specific geometry of these manifolds plays a pivotal role in determining the properties of the vibrating strings, including characteristics such as mass, spin, and electric charge.
In a manner analogous to LLMs, where the next word emerges from a projection within latent spacea high-dimensional mathematical field that encodes relationships of meaning, syntax, and contextan electrons charge may similarly arise as an emergent property derived from the geometry of hidden dimensions in string theory.
Thus, the central theme becomes clear.
The Geometry of Emergence
The dot product in artificial intelligence bears a conceptual similarity to the vibrational projection in string theory. Both processes compress high-dimensional structures into meaningful and observable phenomena.
Traditionally, we tend to perceive intelligence and reality as being constructed from fundamental elementsneurons, bits, particles. However, what if this perspective is misguided? What if these elements are not the foundational building blocks but rather shadowsmanifestations of more profound geometric truths?
In the context of large language models, intelligence is not merely encoded in predefined rules; it emerges organically from structural configurations. Likewise, one could argue that matter itself may originate in a similar fashion. Just as a word forms through the alignment of vectors in latent space, a particle might arise from the synchronization of string vibrations in geometric space. Both meaning and mass represent forms of reductiontwo outcomes of projection.
This observation should not be misconstrued as suggesting that AI equates to physics. Instead, it posits that our models of intelligence and our models of matter may indeed share a common language rooted in high-dimensional geometry and the emergent properties it facilitates.
So What?
Why should we care about these insights? As AI technologies become increasingly integral to our processes of thinking, writing, and reasoning, it is essential to begin viewing them not solely as tools but as portals to understanding. They provide a novel framework for comprehending how meaning emergesnot only in language but also in the very fabric of reality itself.
We may be on the verge of a new epistemological paradigmone in which the boundaries separating thought from matter, language from law, become not only porous but profoundly geometric. And perhaps, just perhaps, a scalar projection is not merely the mechanism by which a machine selects the next word; it may be a glimpse into how the universe communicates.