Advancements in Nanopore Sequencing: A Detailed Exploration of Plasmid Classification Techniques
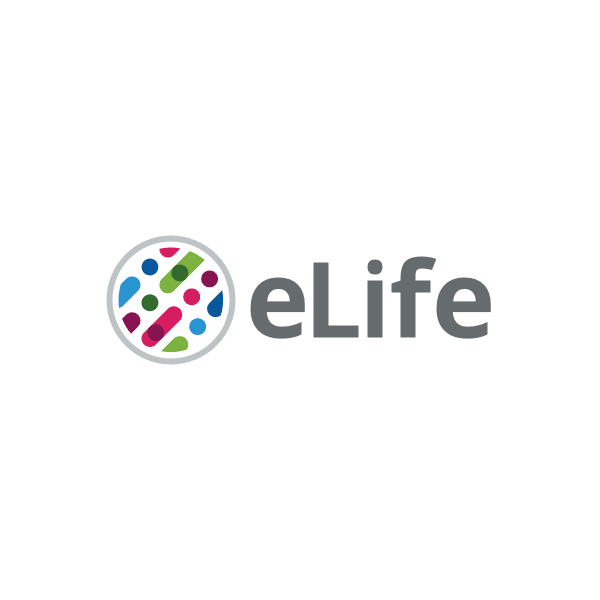
Recent advancements in nanopore sequencing have opened new frontiers in the classification of plasmids, which are small, circular DNA molecules found in bacteria. In a study focused on enhanced methods for analyzing reads from nanopore sequencers, researchers have detailed a refined approach to aligning query sequences against reference plasmids. The initial step involves performing an alignment where the reference plasmid, denoted as pi, is compared against a query sequence qk from each nanopore using specific parameters laid out in a pre-survey algorithm.
The crux of this methodology lies in calculating the normalized alignment score, api, qk, which is assessed by dividing the alignment score by the length of the reference plasmid pi. The assignment of a query sequence to a plasmid, represented as pqk, is determined through the following formula:
pqk = argminpi P api, qk,
where P denotes the set of reference plasmids that were mixed in the analysis. If, however, the score apqk, qk falls below a user-defined threshold, known as score_threshold, this indicates a cutoff for shorter reads, and such readings are excluded to enhance the quality of subsequent analyses. Furthermore, any readings showing identical normalized alignment scores against multiple plasmids are also omitted due to their insufficient information to clearly ascertain their plasmid origin. The procedure additionally discards readings that are significantly longer than the reference plasmid, specifically those more than double the length of pqk, which also exhibit a higher normalized alignment score than 1. This step is crucial in avoiding the misclassification of plasmid multimers.
The results from various plasmid groups are vividly illustrated in Figures 3 and 4. Group I, for instance, showcases multiplex sequencing of six plasmids that exhibit only moderate similarity. In contrast, Groups II through IV consist of two plasmids each that are highly similar to one another. For clarity, its important to note that although plasmids in different figures are labeled similarly (e.g., P1, P2), they represent distinct entities; for example, P1 in Figure 2 is not the same as P1 in Figure 3.
Figure 3a highlights the pre-survey results for Group I, revealing two distinct clusters: one comprising four closely related plasmids (P1 to P4) and another consisting of two (P5 and P6). Each cluster showcases plasmids that share a common vector backbone while possessing different inserts. This illustrates that, despite moderate similarities, multiplexing remains viable owing to their substantial distances exceeding the established quality-oriented cutoff of 20, as depicted in Figure 2c. These six plasmids underwent mixing and were subsequently analyzed as a single sample through nanopore sequencing. Figure 3b serves as a general quality check, depicting the distributions of read lengths and quality scores.
Further analysis of the classified reads revealed distinct read length distributions, underscoring the accuracy of the classification process. The similarity in quality score distributions for reads assigned to each plasmid indicates that each was sequenced with a consistently high quality level. However, it is noted that the quantity of reads was not uniform across each plasmid, which is reflected in the total histogram areas in the read length distribution graphs. The scatter plots of normalized alignment scores presented in Figure 3c serve as a valuable tool for adjusting the score_threshold. Notably, a higher threshold could enhance classification accuracy, though it may subsequently reduce the number of reads assigned to each reference plasmid. Through experimentation, a score_threshold value of 0.5 has been identified as reasonable, allowing users to fine-tune this parameter to optimize data acquisition. For instance, if the total read count is low yet the plasmids are distinctly different, a lower threshold may be advantageous to increase the number of reads advanced to subsequent analysis. Conversely, a higher threshold may be beneficial when dealing with a large number of highly similar plasmids, thereby improving the overall quality of the analysis.
In Figure 4, results from various combinations of plasmids that exhibit high sequence similarity are examined. The primary concern in this scenario is the accurate classification of reads to the respective reference plasmids. The classification of a read to either plasmid is intrinsically linked to the specific regions where their sequences diverge. This is pertinent because errors in regions of identical sequences will affect the normalized alignment score for both plasmids equivalently. Remarkably, accurate classification can be achieved for plasmids differing by just a single base, thanks to the high precision of contemporary nanopore sequencing technologies. To illustrate this, three sets of two plasmids were mixed (set 1: P1 and P2, set 2: P3 and P4, set 3: P5 and P6) with Levenshtein distances of 1, 2, and 3, respectively, and analyzed as a single sample (Figure 4ac). The outcomes after the classification phase are depicted in Figure 4df. Given the near-identical nature of the plasmids, data points predominantly overlap along the y=x line in the scatter plots; however, a magnified view reveals deviations from this line, indicating successful separation of numerous reads.
The researchers conducted a quantitative analysis to estimate the rate of incorrect classification. This involved extracting reads that correspond to areas where the plasmids differ and assessing their classification outcomes. For sets 1, 2, and 3, the respective counts of correctly classified reads stood at 239 out of 303, 262 out of 297, and 2212 out of 2359 reads. The classification breakdowns are illustrated in Figure 4gi, where the top histograms depict the number of reads falling below the defined score_threshold (0.5 in this case), represented in light gray. The minimal numbers in these categories suggest high sequencing quality. The accompanying rotated heatmaps provide an in-depth breakdown of the reads represented in the histograms. Through structured data from these heatmaps, a fitting using the least squares method was performed, wherein the quantity of reads associated with each plasmid and the nanopore error rate were variables. By approximating the probability of base calling errors as consistent regardless of the actual base identity, the results indicated that even plasmids differing by a single base could be classified with a confidence level reflecting an incorrect classification rate of approximately 0.03-1.4%. This ratio diminishes further with increasing Levenshtein distances, translating to lower incorrect classification rates of 0.0074-0.0089% and 0.0143-0.0158% for distances of 2 and 3, respectively. These findings suggest that nanopore base calling errors have a negligible impact on classification accuracy, particularly for pairs of plasmids with distinctions of two or more bases. However, it is recommended to mix plasmids differing by at least two bases, setting a distance_threshold value accordingly, for several reasons: it minimizes the potential effect on consensus quality scores, as the algorithm does not incorporate corrections for low classification confidence, considers the rare instances of elevated error rates for specific sequences, and addresses the risk of unexpected mutations affecting classification.
The post-classification analysis involves aligning each read against its respective reference sequence, followed by a final step to obtain the consensus sequence and quality scores. In this stage, aligned query sequences and their quality scores are integrated using Bayesian analysis, replicating methods previously reported for single nucleotide polymorphism (SNP) detection. Two types of prior information are applied when generating consensus sequences in the analysis software SAVEMONEY: (1) the error rate pertinent to plasmid construction (set arbitrarily during PCR, ligation, or assembly) and (2) the characteristics of nanopore reads, specifically the error rate and quality score distribution per base. For example, if the correct base is identified as A based on 10 readswhere 8 indicate A and 2 indicate Gthe calculation incorporates various scenarios to determine the most probable correct base. Although quality scores from Oxford Nanopore Technologies have not always aligned perfectly with Phred scores, they have become increasingly accurate in recent years, allowing for practical consideration of quality scores as Phred equivalents while accepting minor calculation errors in consensus quality scores.
In conclusion, the process for determining the final consensus base calling and the corresponding consensus Phred score can be articulated through a series of equations grounded in Bayesian statistics. The refinement of plasmid classification techniques using nanopore sequencing not only enhances accuracy but also opens possibilities for more complex biological analysis in the field of genetic research.